Analytics Case Study
Risk Modeling
Our team built, tested, and refined a predictive model that analyzed historical data from multiple sources and compared against current data.
The Problem
Faced with growing numbers of beneficiaries in public assistance programs, and increasing budget constraints, the client – a very large state agency – needed to streamline the eligibility review process so staff could process more applications, with greater speed and accuracy.
Our Solution
Our team built, tested, and refined a predictive model, as proof of concept, that identified changes in beneficiary income that might impact eligibility. Two-Class Logistic Regression and Decision Forest models were built using Microsoft Azure Machine Learning and cross-validated for accuracy.
By analyzing historical data from multiple sources, and comparing against current data, the predictive model could determine the likelihood that income had changed and assign risk scores based on the client’s review criteria. The resulting scores were used to stratify and group cases, based on whether the cases required “No Review,” “Partial Review” or “Full Review.”
Our team demonstrated that its predictive model could accurately calculate risk scores for use in stratifying cases. This process helped to reduce the number of cases that required a manual review, allowing staff to spend more time on cases with greater potential for risk and/or using more senior staff to review high-risk cases. In addition, we demonstrated that the model could provide support for human decision-making and help to better manage the workloads of staff, making them more efficient and effective and, in some cases, automating the entire decision-making process.
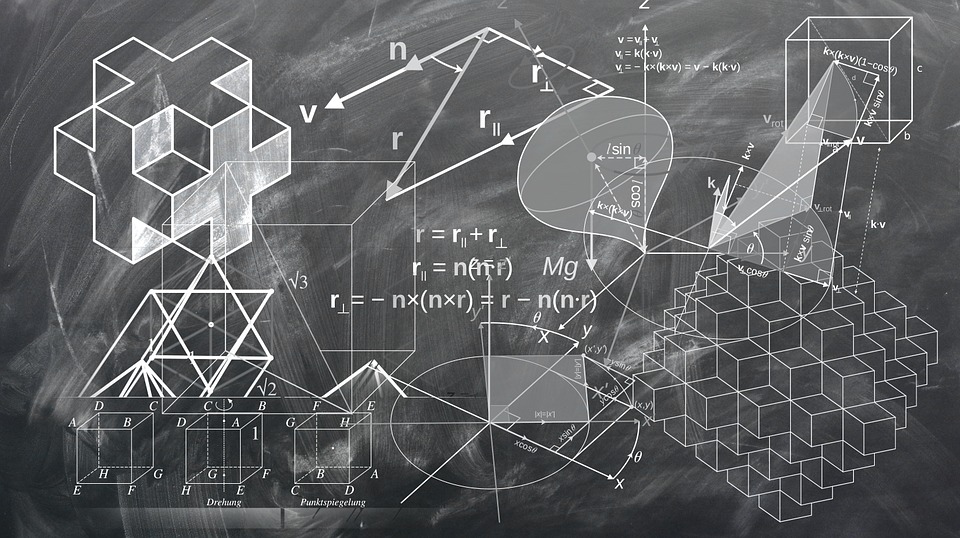
Challenges
- Aligning the predictive model with the client’s review criteria
- Validating data provided by applicants and beneficiaries through independent sources
- Complying with State and Federal regulations
- Meeting stringent privacy and security requirements
- Providing audit functionality
Benefits
- Automated processing of low-risk cases
- Reduced workloads and administrative overhead
- Speedier review process
- Increased efficiency and accuracy
- Increased program integrity
- Significant cost savings to the client